DLA-based compressed sensing for high resolution MR of neuronal tissue
- 1. NeuroSpin, CEA Saclay, Gif-sur-Yvette, France
- 2. University Paris-Sud, Orsay, France
- 3. INRIA Saclay-Equipe DEFI CMAP, Ecole Polytechnique, Palaiseau, France
Introduction: Despite the fact that modern magnetic resonance imaging (MRI) hardware often results in sufficiently high signal-to-noise ratio (SNR) for a single signal average to be acquired, the total experimental time, dictated by the requirement for sufficient k-space coverage, can be extremely long, prohibiting the very high resolution imaging of live biological samples. In such cases compressed sensing (CS) techniques are promising [1]. In this work we present the implementation of CS on a high field preclinical scanner using an under-sampling trajectory based on the diffusion limited aggregation random growth model (DLA) [2] and demonstrate its applicability to high resolution MR studies.
Methods: The first goal of the study was to evaluate the DLA under-sampling method against the standard polynomial sampling pattern [1]. A total of 300 polynomial and 300 DLA trajectories were generated (Fig. 1a) for 11 under-sampling ratios between 0.2 and 0.7. These trajectories were applied to a library of six fully sampled T2 weighted images of buccal and abdominal ganglia of Aplysia californica, acquired on a 17.2 T imaging system. CS image reconstruction was performed using a 3D version of the Sparse MRI toolbox with a total-variation (TV) [1] penalty. To further test the applicability of the proposed method for microscopic imaging, CS 3D images (0.5 under-sampling ratio) of buccal and abdominal ganglia were acquired and compared with fully-encoded data sets (spatial resolution 25μm3).
Results: As seen in Fig. 1b, for under-sampling ratios superior to 0.25, the mean relative errors between fully encoded and CS images are smaller for DLA than for polynomial patterns. Moreover, the DLA method is more stable as the standard deviation of the relative error is smaller than for the polynomial scheme. The CS data sets present higher SNR than the fully encoded acquisitions due to the reduction of noise in the CS reconstructed image. Specifically, measurements performed in the red ROIs (Fig. 1c) resulted in SNRCS=1358/106=12.8 and SNRfully-encoded=1292/138=9.36 respectively. The automatic cell detection performed similarly for CS and fully encoded images, with an identical number of cells detected for abdominal ganglia and one false positive for CS (out of 31 cells) for the buccal ganglia.
Conclusion: The DLA under-sampling scheme introduced here performs better than the standard polynomial approach and it is applicable to high resolution imaging of live neuronal tissue.
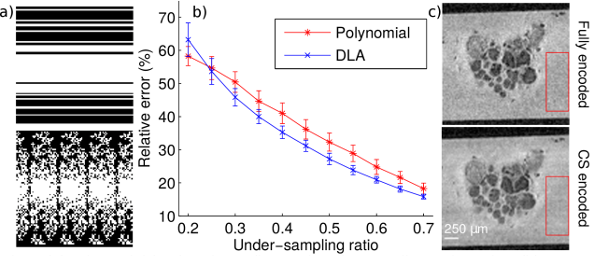